Knowledge Augmented FMs
- SlideDeck: W8-T1-KnowledgeAugmentedFMs.pdf
- Version: current
- Lead team: team-1
- Blog team: team-6
In this session, our readings cover:
Required Readings:
Retrieval-Augmented Generation for AI-Generated Content: A Survey
- https://arxiv.org/abs/2402.19473v1
- The development of Artificial Intelligence Generated Content (AIGC) has been facilitated by advancements in model algorithms, scalable foundation model architectures, and the availability of ample high-quality datasets. While AIGC has achieved remarkable performance, it still faces challenges, such as the difficulty of maintaining up-to-date and long-tail knowledge, the risk of data leakage, and the high costs associated with training and inference. Retrieval-Augmented Generation (RAG) has recently emerged as a paradigm to address such challenges. In particular, RAG introduces the information retrieval process, which enhances AIGC results by retrieving relevant objects from available data stores, leading to greater accuracy and robustness. In this paper, we comprehensively review existing efforts that integrate RAG technique into AIGC scenarios. We first classify RAG foundations according to how the retriever augments the generator. We distill the fundamental abstractions of the augmentation methodologies for various retrievers and generators. This unified perspective encompasses all RAG scenarios, illuminating advancements and pivotal technologies that help with potential future progress. We also summarize additional enhancements methods for RAG, facilitating effective engineering and implementation of RAG systems. Then from another view, we survey on practical applications of RAG across different modalities and tasks, offering valuable references for researchers and practitioners. Furthermore, we introduce the benchmarks for RAG, discuss the limitations of current RAG systems, and suggest potential directions for future research. Project: this https URL
Retrieval-Augmented Generation for Large Language Models: A Survey
- https://arxiv.org/abs/2312.10997
- Large language models (LLMs) demonstrate powerful capabilities, but they still face challenges in practical applications, such as hallucinations, slow knowledge updates, and lack of transparency in answers. Retrieval-Augmented Generation (RAG) refers to the retrieval of relevant information from external knowledge bases before answering questions with LLMs. RAG has been demonstrated to significantly enhance answer accuracy, reduce model hallucination, particularly for knowledge-intensive tasks. By citing sources, users can verify the accuracy of answers and increase trust in model outputs. It also facilitates knowledge updates and the introduction of domain-specific knowledge. RAG effectively combines the parameterized knowledge of LLMs with non-parameterized external knowledge bases, making it one of the most important methods for implementing large language models. This paper outlines the development paradigms of RAG in the era of LLMs, summarizing three paradigms: Naive RAG, Advanced RAG, and Modular RAG. It then provides a summary and organization of the three main components of RAG: retriever, generator, and augmentation methods, along with key technologies in each component. Furthermore, it discusses how to evaluate the effectiveness of RAG models, introducing two evaluation methods for RAG, emphasizing key metrics and abilities for evaluation, and presenting the latest automatic evaluation framework. Finally, potential future research directions are introduced from three aspects: vertical optimization, horizontal scalability, and the technical stack and ecosystem of RAG.
More Readings:
Sora: A Review on Background, Technology, Limitations, and Opportunities of Large Vision Models
- Yixin Liu, Kai Zhang, Yuan Li, Zhiling Yan, Chujie Gao, Ruoxi Chen, Zhengqing Yuan, Yue Huang, Hanchi Sun, Jianfeng Gao, Lifang He, Lichao Sun
- Sora is a text-to-video generative AI model, released by OpenAI in February 2024. The model is trained to generate videos of realistic or imaginative scenes from text instructions and show potential in simulating the physical world. Based on public technical reports and reverse engineering, this paper presents a comprehensive review of the model’s background, related technologies, applications, remaining challenges, and future directions of text-to-video AI models. We first trace Sora’s development and investigate the underlying technologies used to build this “world simulator”. Then, we describe in detail the applications and potential impact of Sora in multiple industries ranging from film-making and education to marketing. We discuss the main challenges and limitations that need to be addressed to widely deploy Sora, such as ensuring safe and unbiased video generation. Lastly, we discuss the future development of Sora and video generation models in general, and how advancements in the field could enable new ways of human-AI interaction, boosting productivity and creativity of video generation.
A Comprehensive Study of Knowledge Editing for Large Language Models
- https://arxiv.org/abs/2401.01286
- Large Language Models (LLMs) have shown extraordinary capabilities in understanding and generating text that closely mirrors human communication. However, a primary limitation lies in the significant computational demands during training, arising from their extensive parameterization. This challenge is further intensified by the dynamic nature of the world, necessitating frequent updates to LLMs to correct outdated information or integrate new knowledge, thereby ensuring their continued relevance. Note that many applications demand continual model adjustments post-training to address deficiencies or undesirable behaviors. There is an increasing interest in efficient, lightweight methods for on-the-fly model modifications. To this end, recent years have seen a burgeoning in the techniques of knowledge editing for LLMs, which aim to efficiently modify LLMs’ behaviors within specific domains while preserving overall performance across various inputs. In this paper, we first define the knowledge editing problem and then provide a comprehensive review of cutting-edge approaches. Drawing inspiration from educational and cognitive research theories, we propose a unified categorization criterion that classifies knowledge editing methods into three groups: resorting to external knowledge, merging knowledge into the model, and editing intrinsic knowledge. Furthermore, we introduce a new benchmark, KnowEdit, for a comprehensive empirical evaluation of representative knowledge editing approaches. Additionally, we provide an in-depth analysis of knowledge location, which can give a deeper understanding of the knowledge structures inherent within LLMs. Finally, we discuss several potential applications of knowledge editing, outlining its broad and impactful implications.
Even More
A Survey of Table Reasoning with Large Language Models
- Xuanliang Zhang, Dingzirui Wang, Longxu Dou, Qingfu Zhu, Wanxiang Che
- https://arxiv.org/abs/2402.08259
- Table reasoning, which aims to generate the corresponding answer to the question following the user requirement according to the provided table, and optionally a text description of the table, effectively improving the efficiency of obtaining information. Recently, using Large Language Models (LLMs) has become the mainstream method for table reasoning, because it not only significantly reduces the annotation cost but also exceeds the performance of previous methods. However, existing research still lacks a summary of LLM-based table reasoning works. Due to the existing lack of research, questions about which techniques can improve table reasoning performance in the era of LLMs, why LLMs excel at table reasoning, and how to enhance table reasoning abilities in the future, remain largely unexplored. This gap significantly limits progress in research. To answer the above questions and advance table reasoning research with LLMs, we present this survey to analyze existing research, inspiring future work. In this paper, we analyze the mainstream techniques used to improve table reasoning performance in the LLM era, and the advantages of LLMs compared to pre-LLMs for solving table reasoning. We provide research directions from both the improvement of existing methods and the expansion of practical applications to inspire future research.
Blog: Retrieval-Augmented Generation for AI-Generated Content: A Survey
Motivation and the RAG Process
Artificial Intelligence Generated Content(AIGC) refers to the texts and code generated by Large Language Model, the images generated by DALL-E and Stable-Diffusion, and video generated by Sora. Besides the recent success of AIGC, it continues to face a number of challenges. For example, it is difficult to maintain up-to-date knowledge for these models, because model training is required in order for the model to generate answers based on new knowledge. In addition, these models suffer from the inability to provide long-tail knowledge, and they are at risk of leaking private training data. Retrieval-Augmented Generation(RAG) serves as a mitigation to these problems, because it has an adaptive data repository. With such data repository, when the new knowledge or long-tail knowledge is included, or when the sensitive private data is encoded, the above challenge can be straightforwardly allievated.
The figure below shows the standard Retrieval-Augmented Generation process. The user’s prompt (in any modalities) is taken as input for both the retriever and the generator. The retriever has access to database and retrieve the data relavent to the prompt for the generator. The generator then takes both the user prompt and the data retrieved as input and eventually generates the results.
Taxonomy of RAG Foundations
The figure below shows the four major categories of RAG.
- Query-Based RAG
- It combines the data retrieved and the user’s prompt as the input for the generator.
- Examples include REALM that uses two BERT for retrieval and generation, and APICoder for text to code tasks.
- Latent-Representation Based RAG
- This line of methods allows the generator to deal with the latent representation of retrieved data.
- FiD is a common technique used that process the retrieved data by an encoder individually.
- The benefit of such technique is that it can generate answers after fusing multiple paragraphs in the latent representation.
- Logits-based RAG
- The retrieved data is incorperated in the logits during the decoding process.
- Some examples includes kNN-LM that augments LM with k-nearest neighbour search and TRIME.
- Speculative RAG
- This category of RAG decide when to use retriever to augment the generation process to save inference time.
Taxonomy of RAG Enhancements
The performance of RAG can be further enhanced by the following techniques shown in the below figure.
- Input Enhancement can be done in the following two ways:
- Query Transformation: The user’s input prompt can be enhanced by modifying the query.
- Data Augmentation: the retrival database can exclude irrelavent data before making the retrieval.
- Retriever Enhancement
- Recursive Retrieve: a query is splitted into smaller pieces and result is combined by multiple retrievals.
- Chunk Optimization: the size of the chunk is adjusted to achieve better retrieval results.
- Some other technniques include Finetune Retriever, Hybrid Retrieve, Re-ranking and Meta-data Filtering.
- Generator Enhancement
- In a RAG system, the generator is the “upperbound” of the performance, and it is enhance by methods such as Prompt Engineering, Decoding Tuning and Finetune Generator.
- Result Enhancement
- In some cases, it is possible to rewrite the output in order to improve the performance.
- RAG Pipeline Enhancement
- Within the RAG pipeline, the model can decide when to perform retrieval to obtain the best performance.
- An iterative retrieval process may also further improve the performance.
Taxonomy of RAG Applications
RAG is a general purpose method that can be effectively applied in different domains. The figure below shows the areas of its application, ranging from question answering, code generation, to text-to-3D and drug discovery.
Sora: A review on Background, Technology, Limitations, and Opportunities of Large Vision Models
What is Sora?
Sora is a text-to-video generative AI model, released by OpenAI in February 2024. The model is trained to generate videos of realistic or imaginative scenes from text instructions and show potential in simulating the physical world. Figure below is an example of the input and output of Sora.
What can Sora do?
The implications of Sora extend far beyond mere video creation, offering transformative potential for tasks ranging from automated content generation to complex decision-making processes. Figure below is an overview of practical deployment scenarios.
History of Generative Video
Overview
Sora is a diffusion transformer with flexible sampling dimensions as shown in figure below. It has three parts:
- A time-space compressor first maps the original video into latent space.
- A ViT then processes the tokenized latent representation and outputs the denoised latent representation.
- A CLIP-like conditioning mechanism receives LLM-augmented user instructions and potentially visual prompts to guide the diffusion model to generate styled or themed videos.
Data Pre-processing
Variable Durations, Resolutions, Aspect Ratios
Sora can generate images in flexible sizes or resolutions ranging from 1920x1080p to 1080x1920p and anything in between.
Sora is trained on data in their native sizes which significantly improves composition and framing in the generated videos. The comparison between Sora and a model trained on uniformly cropped square videos demonstrates a clear advantage as shown in figure below. Videos produced by Sora exhibit better framing, ensuring subjects are fully captured in the scene.
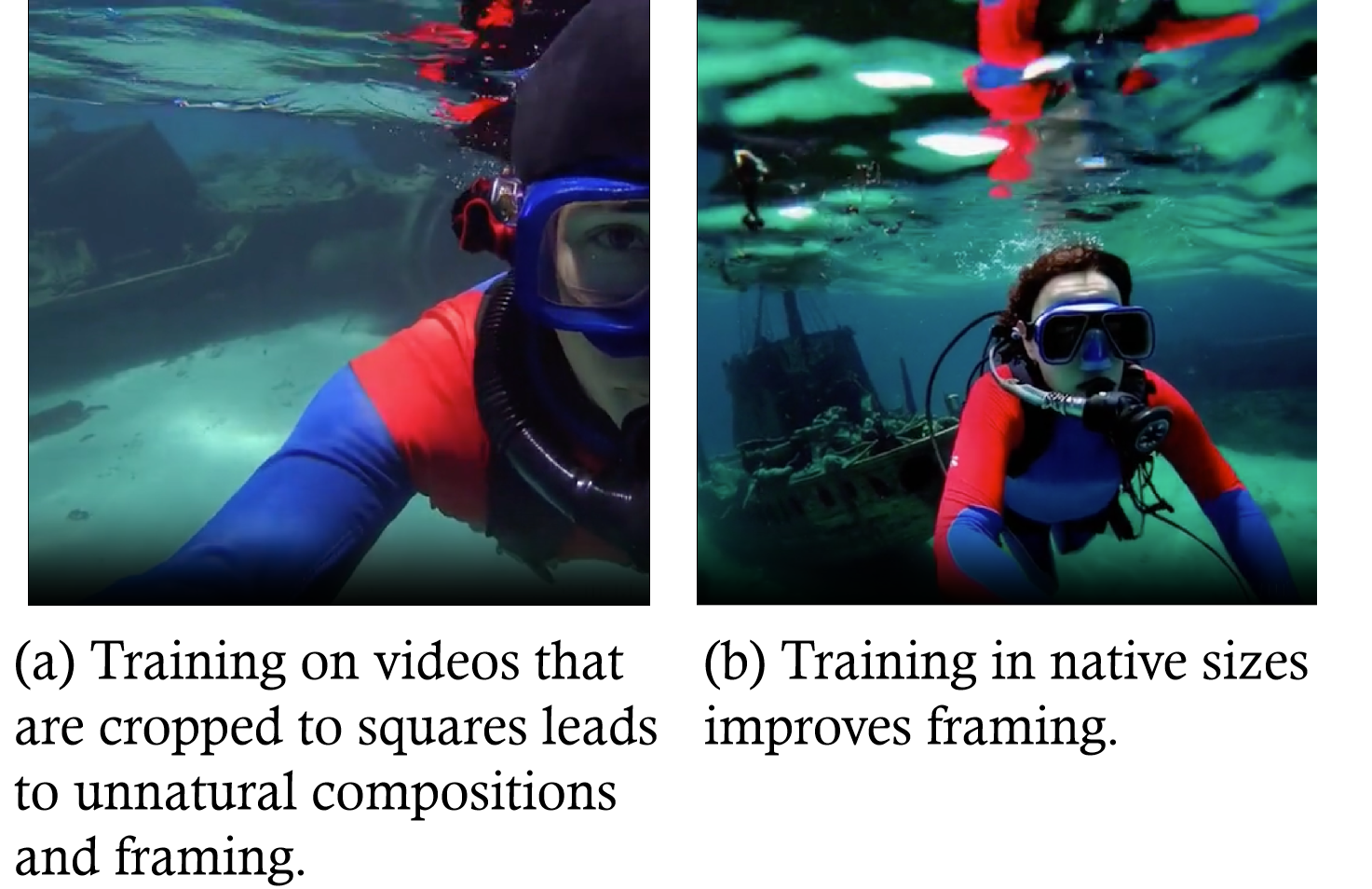
Unified Visual Representation
To effectively process diverse visual inputs including images and videos with varying durations, resolutions, and aspect ratios, Sora patchifies videos by initially compressing videos into a lower-dimensional latent space, followed by decomposing the representation into spacetime patches, as shown in the figure below.
Video Compression Network
Sora’s video compression network (or visual encoder) aims to reduce the dimensionality of input data. It is typically built upon VAE or Vector Quantised-VAE (VQ-VAE). To solve the problem that it is challenging for VAE to map visual data of any size to a unified and fixed-sized latent space, there are two implementations.
- Spatial-patch Compression: Transforming video frames into fixed-size patches
- Spatial-temporal-patch Compression: Consider spatial and temporal dimensions of data and captures changes across frames. Compared with pure spatial-pachifying, 3D Convolution is utilized to achieve spatial-temporal-patch compression as shown in figure below.
Spacetime Latent Patches
A remaining concern in compression network part is: How to handle the variability in latent space dimensions (i.e., the number of latent feature chunks or patches from different video types) before feeding patches into the input layers of the diffusion transformer.
Patch n’ pack (PNP) is a possible the solution. PNP packs multiple patches from different images in a single sequence as shown in figure below.
Modeling
Image Diffusion Transformer
DiT and U-ViT are among the first works to employ vision transformers for latent diffusion models. DiT employs a multi-head self-attention layer and a pointwise feed-forward network interlaced with some layer norm and scaling layers. DiT incorporates conditioning via adaptive layer norm (AdaLN) with an additional MLP layer for zero-initializing, which initializes each residual block as an identity function and thus greatly stabilizes the training process.
Video Diffusion Transformer
Imagen Video developed by Google Research, utilizes a cascade of diffusion models, which consists of 7 sub-models that perform text-conditional video generation, spatial super-resolution, and temporal super-resolution, to transform textual prompts into high-definition videos as shown in figure below.
Some points that worth noting:
- Imagen architecture utilizes 3D U-Net architecture with temporal attention mechanisms and convolution layers to maintain the consistency and flow between frames.
- U-Net is not necessary for performance of traditional diffusion architecture.
- Adopting transformer instead of U-net is more flexible since it can allow for more training data and more model parameters.
Language Instruction Following
Another question is: How does Sora follow user instructions?
- DALLE-3 uses Contrastive Captioners (CoCa) to train an image captioner with CLIP jointly with a language model objective.
- Mismatch between user prompts and image descriptions pose a problem.
- LLMs are used rewrite descriptions into long descriptions.
- Similar to DALLE-3, Sora uses video captioners to trained to create detailed descriptions for videos.
- Little description
- Likely uses VideoCoCa, which is build on top of CoCa.
Prompt Engineering
Text Prompt
Prompt engineering can leverage model’s natural language understanding ability to decode complex instructions and render them into cohesive, lively, and high-quality video narratives. Figure below is an example.
Image Prompt
An image prompt serves as a visual anchor for the to-be-generated video’s content. The use of image prompts allows Sora to convert static images into dynamic, narrative-driven videos by leveraging both visual and textual information. Figure below is an example
Video Prompt
Work like Moonshot and Fast-Vid2Vid demonstrate that a good video prompt requires being specific and flexible so that the model gets a clear direction and objectives.
-
Trustworthiness
- Safety Concern
- Large multi-modal models are vulnerable to adversarial attacks due to their high dimensional nature and ability to take visual input.
- Hallucination is a problem.
- Fairness and Bias
- How to mitigate bias in Sora from training data and make the model operate fairly?
- Privacy preservation
- Can Sora protect user data?
- Alignment
- It is important to ensure human intentions and model behavior are aligned.
- RLHF used in LLMs, what will be done for Sora?
- Recommendations for Future works:
- Integrated Protection of Model and External Security.
- Security Challenges of Multimodal Models.
- The Need for Interdisciplinary Collaboration.
Limitations
- Lacks in physical realism, especially complex scenarios.
- Spatial and temporal misunderstandings.
- Limits in Human-computer interaction.
- Usage limitation.
A Comprehensive Study of Knowledge Editing for Large Language Models
Large Language Models (LLMs) are the maestros of modern text generation, strikingly mimicking the nuances of human communication. Yet, their brilliance comes with a challenge – the heavyweight computational cost of their expansive learning capacity. As our world shifts, so must our models; their knowledge is a race against time, continuously needing updates to stay accurate and relevant. Enter the realm of knowledge editing – a promising avenue where the agility of model modifications is not just a desire but a necessity for applications demanding precision post-training. This paper journeys through the emerging landscape of knowledge editing techniques, offers a fresh benchmark for evaluating their efficacy, and invites us to peer deeper into the cognitive framework of LLMs, setting the stage for innovations with the groundbreaking EasyEdit framework. We stand on the cusp of an era where the adaptability of AI could redefine its role across industries.
Knowledge Editing
Efficiently modify LLMs’ behaviors within specific domains while preserving overall performance across various inputs. For an original model 𝛳, knowledge k and knowledge editing function F, the post-edited model is defined as,
- Knowledge Insertion
- Knowledge Modification
- Knowledge Erasure
Benchmark Data: KnowEdit
6 datasets on knowledge editing are curated. These encompass a range of editing types, i.e., fact manipulation, sentiment manipulation and hallucination generation.
Knowledge Editing Evaluation
- Edit Success
Also termed as Reliability. It is the average accuracy of the edit cases
- Portability
Whether the edited model can address the effect of an edit
- Locality
The edited model should not modify the irrelevant examples in out-of-scopes
- Generative Capacity
Generalization ability of the model after editing. Also, termed ‘fluency’.
Error and Case Analysis
Limitations of Knowledge Editing
- The underlying mechanism of Transformers is opaque. Therefore, it is unclear whether or not the existing knowledge editing methods are truly successful.
- Defining the boundaries of the influence of knowledge editing is challenging. It was compared with neurosurgery, where the assessment of the impact of any modifications is complex.
- Keeping pace with the dynamic and fluid nature of knowledge.
A Survey of Table Reasoning with Large Language Models
Introduction to Table Reasoning
Table reasoning aims to generate accurate answers from tables based on users requirements. And table reasoning task improves the efficiency of obtaining and processing data from massive amounts of tables.
The Rise of LLMs and their Advantages
Traditional methods relied on rule-based systems or neural networks. With LLMs’ vast knowledge and language understanding capabilities, LLMs excel at table reasoning.
There are some key advantages of LLMs in Table Reasoning:
-
Instruction following ability benefits structure understanding
-
Step-by-step reasoning capability benefits schema linking
-
Reduced annotation requirements
Techniques for Improving Performance in LLM era
The authors proposed some techniques for improving performance in LLM era:
-
Supervised Fine-Tuning
-
Result Ensemble
-
In-Context Learning
-
Instruction Design
-
Step-by-Step Reasoning
For Supervised Fine-tuning:
-
Fine-tuning LLMs on annotated data to enhance reasoning capabilities
-
Using pre-existing datasets or manually labeled data
-
Leveraging distilled data generated by other LLMs
-
-
In the LLM era, instruction-based and multi-task data fine-tune models for better generalization
For Result Ensemble:
-
Obtaining diverse results by varying prompts, models, or random seeds
-
Selecting the most suitable answer through scoring or verification
-
Compared to pre-LLM methods, LLMs can generate diverse results more effectively, often by simply changing instructions, unlike pre-LLM methods requiring aligned fine-tuning and inference instructions.
For In-context Learning:
-
Leveraging LLMs’ ability to generate expected answers using suitable prompts
-
In-context learning capability of LLMs allows flexible adjustment of prompts suitable for different questions without further fine-tuning
-
Reduces labeling overhead while enhancing performance
One Example of In-context Learning:ODIS
-
ODIS
-
Ontology-Guided Domain-Informed Sequencing
-
using in-domain demonstrations to enhance model performance by synthesizing in-domain SQL based on SQL similarity
-
The aboving figure shows an example prompt of 2-shot in-domain text-to-SQL
Two in-domain demonstrations are present prior to the test question
For Instruction Design:
-
Utilizing LLMs’ instruction following ability
-
Instruction design involves instructing LLMs to complete decomposed sub-tasks for table reasoning.
- Modular decomposition: Breaking tasks into sub-tasks (DATER)
One Example of Instruction Design: DATER
(Decompose evidence And questions for effective Table-basEd Reasoning)
For Step-by-step Reasoning:
-
Solving complex tasks by incorporating intermediate reasoning stages
-
Techniques like Chain-of-Table
-
Decomposing questions into simpler sub-questions or predefined operations
-
Differs from modular decomposition which breaks tasks into widely different sub-tasks.
-
One Example of Step-by-step Reasoning: Chain-of-Table
Future Research Directions
-
We can focus on improving table reasoning performance:
-
Supervised Fine-Tuning: Establishing Diverse Training Data
-
Result Ensemble: Sampling Results More Efficiently
-
In-Context Learning: Optimizing Prompts Automatically
-
Instruction Design: Automatically Refining Design with Verification
-
Step-by-Step Reasoning: Mitigating Error Cascade in Multi-Step Reasoning
-
-
We can focus on expanding practical applications:
-
Multi-Modal: Enhancing Alignment between Image Tables and Questions
-
Agent: Cooperating with More Diverse and Suitable Table Agents
-
Dialogue: Backtracking Sub-tables in Multi-turn Interaction
-
Retrieval-Augmented Generation: Injecting Knowledge Related to Entities
-